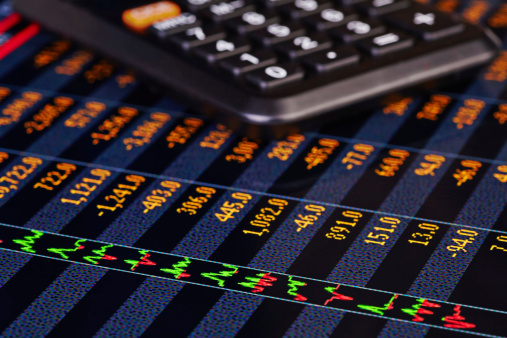
Policymakers should use statistical prediction to analyze social trends and prevent crises before they strike.
Lawmakers are perpetually reactive. They spend years trying to develop fixes to past problems, in the hope that history will not repeat itself. For example, although more than five years have passed since Lehman Brothers filed for bankruptcy, financial regulators are still working to complete all the rulemaking called for under the Dodd-Frank Act. As with the Sarbanes-Oxley Act before it, the architects of Dodd-Frank’s regulatory structure hang their hopes at preventing future crises on massive legislative and rulemaking projects that often extend for years. But too few policymakers and scholars have asked whether a singular focus on preventing the past from repeating itself is the best use of regulatory resources. Rarely do they challenge the common assumption that “never again” should dominate the policymaking agenda.
The key questions are: What will give rise to the next crisis? What type of activity necessitates regulatory intervention to prevent the next economic disaster from occurring? The way to predict where the next disaster might emerge is not to look to the past but instead to identify what large numbers of people are doing today. Market failures lead to crises when they occur repeatedly across large segments of the population. For the housing bubble to form, many homeowners had to act as if home prices could never fall.
Of course, not every activity needs regulatory intervention. The vast majority of individual activities cause no systemic harms and are best left untouched. But when many market actors start engaging in the same risky activity—when a social trend emerges—a shrill “miner’s canary” should alert regulators to consider intervening if the activity has detrimental consequences for society as a whole.
It is now possible to identify the popularity of actual ideas, such as engaging in real estate speculation. The Google Ngram dataset contains 468 billion words, a sample of all English-language books published in the United States each year, which allows policymakers and analysts to measure ideas in society by performing quantitative analysis of textual data. As the words in published books reflect the ideas that people consume, newly popular phrases reveal emerging social trends at a level of specificity that should enable a prompt and effective regulatory response before a crisis unfolds.
To take a concrete example, a particular form of housing speculation known as “flipping” properties became very popular in the years immediately prior to the housing crisis of 2007-08.“Flipping” involves signing a contract of sale to purchase a home, immediately reselling the home to another buyer at a slightly higher price, and arranging the second closing to occur at the same time as the first or shortly thereafter. Flipping properties is a blatant form of speculation that succeeds only if housing prices are generally stable or rising.
What is striking is that despite Federal Reserve Chairman Alan Greenspan’s claim in June 2005 that housing speculation was “difficult,” the popularity of the term “flipping properties” was skyrocketing at the time, undergoing a ten-fold increase in the frequency of its occurrence in books published each year from 2002 to 2006.
As the graph shows, the frequency of “flipping properties” jumped from around 100 occurrences in books published in 2002 to 1,000 in books published in 2006. Regulators should pay attention to ideas like these that “spike” in popularity because they reflect emerging social trends that may necessitate regulatory intervention.
Furthermore, it is possible to identify these ideas by allowing the data to “speak for itself.” Social trends can be detected by sorting phrases by frequency, not by conducting predetermined searches. Newly popular ideas can also be automatically linked to specific administrative agencies based on their regulatory mandates. For example, the words associated with two leading causes of the 2008 financial crisis—“subprime lending” and “credit default swaps”— saw similar sharp upswings in popularity from 2003-2005 and were within the top ten credit-related topics necessitating the attention of the Federal Reserve System during those same years. Wider use of empirical methods of analyzing textual data facilitates can help translate emerging social trends into normative mandates for regulatory agencies.
Some might worry about such techniques finding false positives, but the ability to link the universe of textual data to specific regulatory agencies helps suppress this potential concern. Regulators can distinguish the wheat from the chaff by beginning with textual terms that are known to be associated with each agency’s regulatory mandate, then identifying terms that are likely to be conceptually related. The implications are two-fold. First, regulators need not know what to search for in advance because related ideas can be automatically detected using textual data. Second, irrelevant topics that explode in popularity can remain suppressed because they are not conceptually related to any agency’s regulatory mandate.
Of course, any predictive analysis will have some degree of noise. Social trends will not perfectly predict the need for regulatory intervention. But any improvement in accurately anticipating what may matter tomorrow is better than simply reacting to yesterday’s crisis.
If regulators would start to rely on real-time systems that analyze social trends based on live, streaming data, it will be possible to shorten the well-known “policy gap” between the time when the need for regulation emerges and when corrective action is taken. While some “black swan” events will remain impossible to predict, a real-time regulatory system that analyzes social trends would go a long way toward ensuring that regulatory policy proactively adapts to an evolving society.