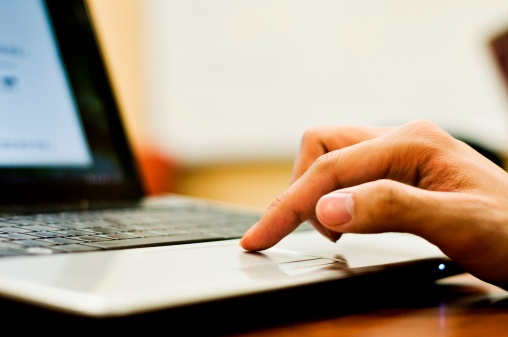
Answering key questions about regulation requires better data.
How much should governments regulate? Economists attach huge significance to this question because the difference between rich and poor countries can be affected by variation in government quality, especially the extent to which the legal system protects property and encourages innovation and investment. But answering this question has been difficult due to limitations both in economic theory and available data. We have created a new dataset that can help overcome the limits in available data.
Admittedly, modern econometric methods have in recent decades allowed economists to advance significantly their ability to answer questions about governmental regulation. Researchers can propose sophisticated responses to questions about the effects of specific laws—e.g., what were the employment consequences of Germany’s Renewable Energy Act?—and thereby help provide well-reasoned bases for future policy decisions.
Yet data limitations make it difficult if not impossible to answer more general questions about regulation. The key ingredient for the empirical analysis of regulation is numerical data, such as profits and costs. But regulation is typically measured in non-numerical, categorical terms.
Researchers use non-numerical data when categories cannot be ranked; for example, location or sector are typical non-ordinal, categorical variables. However, using categorical data forces researchers to narrow the scope of their analysis because these data constrain the researcher when the data exhibit gaps, which they always do. In contrast, numerical variables, such as unemployment or prices, allow researchers to cover gaps by interpolating or extrapolating.
To be more concrete, consider a researcher estimating the effect of mortgage rates on house prices—both numerical variables. According to the data on the Federal Reserve website, mortgage rates have varied substantially since 1971, but the data have gaps: mortgage rates have, for example, sometimes been exactly 3.60% and sometimes exactly 3.68%, but they have never been anything in between. Nor have they ever fallen below 3.35% or exceeded 18.45%. By interpolating and extrapolating, however, we can still make educated guesses about what happens to housing prices when mortgage rates fall within these gaps. For example, a reasonable guess about what happens when rates are 3.64% is that they are halfway between what happens at 3.60% and 3.68%. In this sense, the data’s numerical nature permits the researcher to expand the scope of her analysis beyond the range of the original data.
On the other hand, if the researcher wants to look at the effect of a state jurisdiction—a categorical variable—on something like housing prices, and if she is missing data on 20 states, then she has no reasonable basis for drawing conclusions about those missing states. She could “geographically” interpolate or extrapolate by, for example, assuming that house prices in South Dakota are halfway in between those in North Dakota and Nebraska (its northern/southern neighbors), but that requires a much larger leap of faith. That is why categorical data are less helpful than numerical data.
Government regulations have, at least until recently, been treated as non-ordinal, categorical variables. As a result, it has made little sense to say something like “mining is three times more regulated than farming.” Even if indirect cardinal measures might be available, such as compliance expenditures, they can be misleading proxies for the degree of regulation because they measure more than one thing—both the extent of regulation as well as the costs to comply with or enforce that regulation. For example, in your office, your employer might impose the same constraints on the content of your speech and your email. Yet it is cheap to enforce the email constraints by using software, while enforcing the same constraints on oral speech may require spending huge amounts on proving someone said something inappropriate. If we used enforcement expenditure as a gauge of the degree of regulation, we may incorrectly infer a higher degree of “regulation” on speech than on email, even though the same standard applies to both.
Consequently, existing regulation research—while being valuable—usually has answered only narrow questions, such as, “What was the effect of the Obamacare on unemployment?” or “How do state variations in political affiliation affect the incidence of concealed carry laws?” In both of these examples, more ambitious studies would seek to examine questions that call for a cardinal representation of regulation in general: “What is the effect of regulation, writ large, on unemployment?” or “How does political affiliation in general affect the level of regulation?”
As a complement to empirical work, economists often call for the use of “theory.” This means creating a simplified model of the primary actors, their choices, and the associated incentives, sometimes with the aim of informing the econometric model to be used. Economists often rely on theory because data limitations restrict the ability of purely empirical approaches to deliver definitive conclusions.
In the context of regulation, theory sets the stage for much of the controversy over government intervention. According to the British economist Arthur Pigou, markets can malfunction due to a variety of market failures, such as monopoly power or externalities. In these cases, a sufficiently informed and benevolent policymaker can deploy regulation to enhance societal welfare, such as by adopting anti-trust or environmental laws.
Of course, economist Ronald Coase showed that Pigovian-motivated regulation might be rendered redundant by the organic desire of the affected actors to resolve market failure themselves, through a decentralized, multilateral bargain, aided by courts in the case of disputes. For example, residents concerned about local crime may choose to form a neighborhood watch rather than rely on government intervention. While the affected parties often have the strongest incentive to do something about a market failure, the large numbers may make it impractical: it would be difficult, for instance, to get all the residents of a suburb to bargain and reach a consensus on the optimal level of noise pollution. Hence, economic theory suggests that when “transactions costs” are prohibitive, Pigovian style government regulation may be an efficient alternative.
Economist George Stigler took a more skeptical view of government motives. He argued that the Pigovian model—even one that accepts Coasian insights—assumes benevolent policymakers, when in practice regulations may in fact reflect policymakers’ personal agendas. In the case of “regulatory capture,” leading figures in the regulated industry co-opt the regulator, resulting in regulations that serve the interests of industry leaders at the expense of the industry’s smaller players, potential entrants, or other industries. For example, U.S. car manufacturers might convince the government to impose a tariff on car imports to their benefit and at the expense of U.S. consumers. Historically, there are examples of policymakers taking things a step further and regulating for their own direct benefit, such as when a medieval baron erects a barrier across a river and charges travelers a toll. A hypothetical, modern incarnation would be a government passing stringent safety standards but allowing private actors to obtain discretionary exemptions in exchange for favors, such as financial kickbacks.
Good intentions are one thing, but according to economist Sam Peltzman, good information is another. Whether benevolently-conceived or otherwise, regulations can backfire because regulators lack full information about the future consequences of the rules they adopt. For example, poor design and foresight meant that the Endangered Species Act motivated landowners to “shoot, shovel, and shut-up”—discreetly killing endangered species, rather than protecting them.
Who is right: Pigou or Stigler and Peltzman? Theory only goes so far in answering that question. Because there are sound reasons to anticipate both good and bad consequences from regulations, the burden shifts to empirical research. To refine our knowledge of the causes and consequences of government regulations, we must therefore develop better strategies for empirical inquiry.
As we noted at the outset, the inability to quantify regulation in numerical terms has limited researchers’ ability to generalize about regulation. This is where a new dataset we developed, RegData, can help. RegData is an attempt to loosen the ties that bind regulatory scholars. It is the first database that provides users with an industry-level panel of U.S. federal regulation, turning regulation from a non-ordinal, categorical variable into a numerical one. RegData, which currently covers the period 1997-2012, is produced using custom-made text analysis software to measure, in numerical terms, how restrictive federal regulations are and which industries regulations are most likely to affect. Through RegData analyses, for example, researchers will now be able to compare the level of regulation in U.S. fishing in 2001 to the level of regulation in U.S. fishing in 2009.
In a separate essay, we explain more about how RegData is constructed and what potential it holds. Suffice it to say, with the kind of numerical data about regulation that RegData provides, researchers will be able to study a wider array of questions about regulations’ causes and consequences—and perhaps finally begin to narrow the field’s theoretical divide.
This essay is the first of two by the authors explaining why and how they have created their new dataset, RegData.