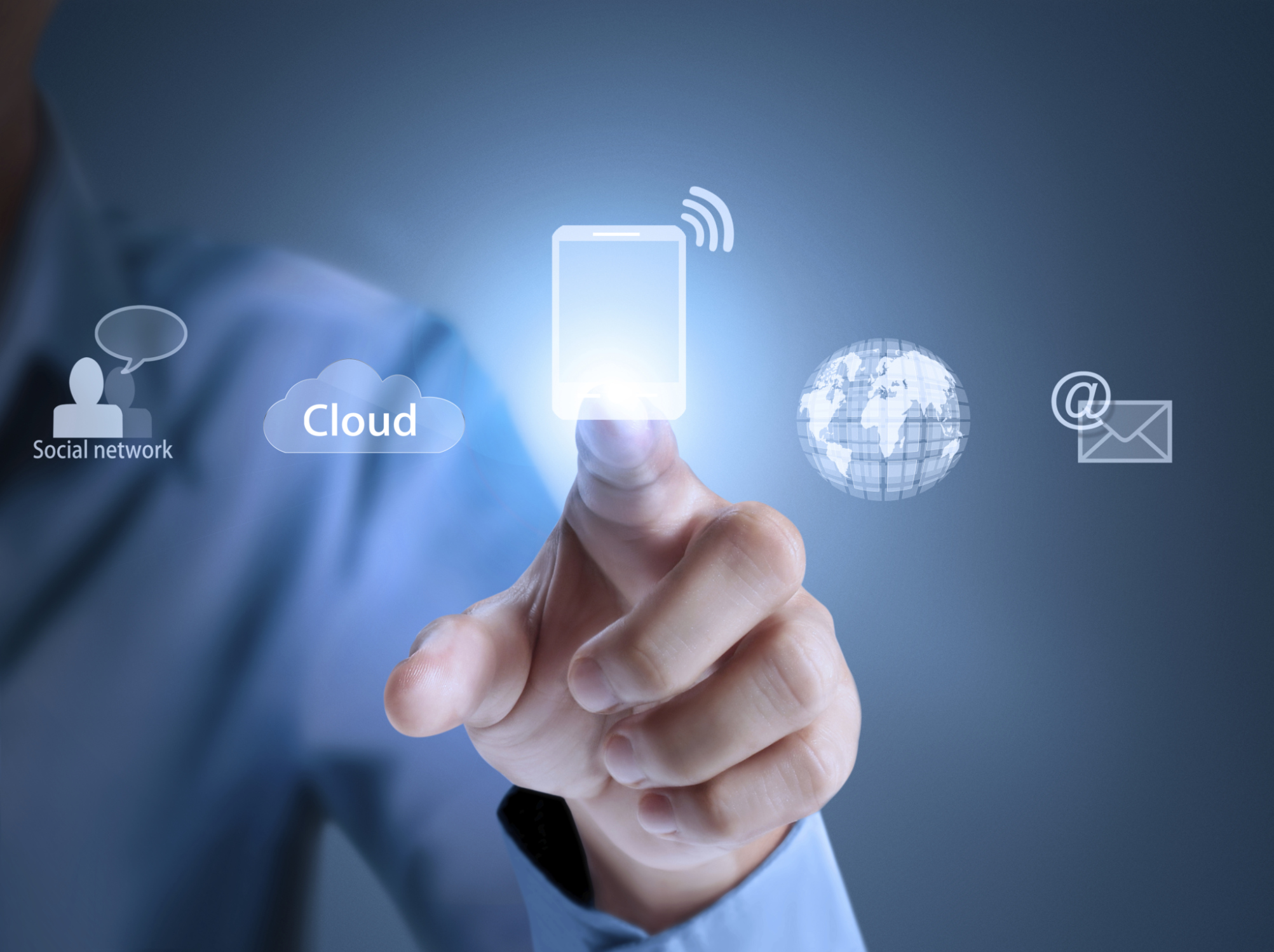
An economy increasingly based on algorithms will need a more sophisticated government, too.
These days, you no longer need to be part of the wealthy elite to access a car service. Nor do you need to sleep in a cramped hotel room to save money on accommodations. Netflix knows just the movie you would love to watch next, and Google is developing a car that will drive itself. Even certain medical treatments can be customized to your genes.
In a paper recently released by the Ewing Marion Kauffman Foundation, Cary Coglianese, a professor at the University of Pennsylvania Law School, argues that these innovations make up a new “optimizing economy.”
Coglianese points out that such innovations and other similar entrepreneurial success stories are not merely additions to the economy; rather, they actually represent optimizations “of existing resources, goods, and services.” And this optimizing economy, he says, requires an equally “optimizing government” to oversee it. In other words, the public sector must keep up with the private sector’s fast-paced developments by fostering its own innovations.
Coglianese breaks down the optimizing economy into three primary characteristics:
- customization;
- the reliance on machine learning (such as when Google analyzes your search history via algorithm to predict what you might be interested in seeing); and
- the better use of distributed resources.
In these three ways, the optimizing economy “contextualizes” in order to match resources better to the nuanced needs of customers, patients, investors, and other economic actors, Coglianese says.
Coglianese argues that the very thing that makes optimization successful – its hyper-contextualization – will cause problems for the government. He points to lawmaking, for example. Laws are meant to be general, and the government aims for standardization. The goal is for everyone to be treated the same. But this creates, writes Coglianese, an “optimization mismatch” between the public and private sectors.
The optimizing economy can create other challenges for government, as well. First, says Coglianese, because the optimizing economy is so new, it is hard to determine what risks exist for different products and services. Second, he argues, it may be difficult to determine if risks exist at all. As products and services become more specific, more precise monitoring is required. Think how precise the manufacturing of a medication that is customized to your genes must be. This precision in itself, argues Coglianese, “makes the government’s job in overseeing product quality that much harder.”
So how can the government keep up with and regulate the optimizing economy?
Coglianese offers several ideas.
First, he says, the government needs to retain and attract employees who can understand complex data analytical processes. Only then will regulatory agencies be able to monitor and respond to issues like those that may arise from the use of machine learning in the private sector.
Second, the federal government needs to improve its technological resources and capabilities. In addition to updating its computer systems, Coglianese suggests that the federal government “combine databases across the federal government and use machine learning to make regulation and other governmental functions smarter.” In fact, says Coglianese, recent research at the Penn Program on Regulation shows that applying machine learning across different datasets can help government regulators more efficiently use their limited resources.
In the end, Coglianese warns against the government making two mistakes when overseeing this new economy. It must not turn a blind eye to potential risks associated with the new, optimizing economy, but also it must not stifle innovation. In order to succeed at becoming an optimizing government, says Coglianese, governmental “action must become smarter than ever before.”