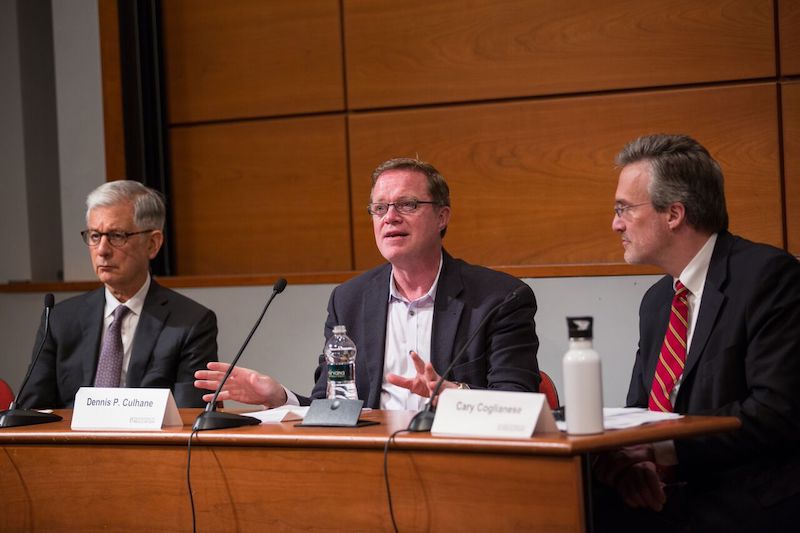
Experts explain how algorithms can aid government health and welfare work.
Pittsburgh residents have long counted Mr. Rogers Neighborhood and McDonald’s Big Mac burgers as among the most famous innovations originating from their city. Today, Pittsburgh has another innovation to trumpet: its surrounding county government has digitized its records and is now using big data analysis to improve health and human services.
Allegheny County, which encompasses Pittsburgh, first integrated its public records nearly twenty years ago. In 2016, officials further modernized services by adopting machine learning to help identify children at risk of injury and death because of unsafe living situations.
At a workshop held earlier this year at the University of Pennsylvania Law School, a trio of policy experts discussed the big data developments in Allegheny County as well as other efforts to build algorithmic decision-making into government services more broadly.
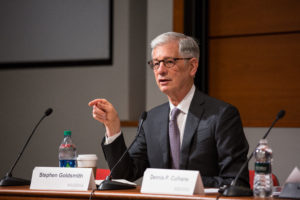
Professor Stephen Goldsmith responds to a question during the workshop.
Dennis Culhane, the Dana and Andrew Stone Professor of Social Policy at the University of Pennsylvania, briefed workshop attendees on the origins and outcomes of Allegheny County’s new child risk assessment screening tool facilitated by machine learning.
Before Allegheny County started using machine learning, child services workers would use intake forms to decide whether to open an investigation into a child’s living circumstances. Tragically, however, human error meant four out of every five fatalities occurred among kids who had been inadvertently screened out of the system, Culhane explained.
Allegheny County’s new system uses a predictive model to comb government records—from sectors including health, justice, and employment—to generate a risk score. This score aids case workers in deciding which child welfare reports require further investigation. Although further research is underway, Culhane reported early analysis showing that the use of machine learning has dramatically reduced false positives and improved accuracy in identifying children in high-risk homes.
Allegheny County should inspire other state and local governments, too many of which have clung to outmoded attitudes and practices about data use, remarked Stephen Goldsmith, another speaker at the workshop. Goldsmith, a professor at the Harvard Kennedy School, previously served as the Mayor of Indianapolis and Deputy Mayor of New York City. Goldsmith argued that in response to the corruption rampant in machine politics of the nineteenth and early twentieth centuries, policies evolved to take discretion away from government workers. Yet lack of decision-making authority dissuades innovation, he continued—it means public employees are left to complete “commodity” tasks and fill out forms, rather than improving society.
Goldsmith advocated more efforts like Allegheny County’s, where commodity tasks and performance monitoring can be turned over to machine learning, freeing up public servants to carry out the public spirited mission of their jobs. He argued that more than 60 percent of 311 calls could be handled by robots. Initial screening by algorithms would allow emergency call center employees to engage more productively with the callers who actually require human attention.
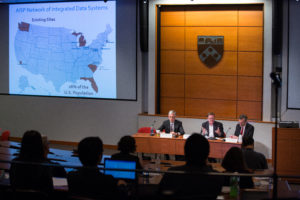
Workshop attendees listen as the panelists discuss efforts to use algorithms in providing government services.
Or consider street lights. Goldsmith asked, Why should a city worker wait until a citizen complains about a burned out bulbs when a simple sensor can transmit the information to a central office in advance of the outage?
Goldsmith and Culhane acknowledged that “institutional inertia” and legal concerns have slowed many localities’ efforts to improve decision-making using big data and machine learning. But the workshop’s final presenter, Cary Coglianese, a professor at the University of Pennsylvania Law School, where he also directs the Penn Program on Regulation, pointed out that algorithmic tools can fall “comfortably” into existing administrative and constitutional legal frameworks.
Drawing on a recent paper he coauthored with David Lehr, as research affiliate with the Penn Program on Regulation and a student at Yale Law School, Coglianese noted that most areas of agency discretion are already insulated from judicial review. This protection makes decisions like which restaurants to inspect or which street lamps to change ripe for efficiency and accuracy improvements using algorithms, Coglianese observed.
Coglianese also argued that machine learning analysis will be treated by the legal system much like other analysis. Regulators already use methods such as cost-benefit analysis to inform decisions, Coglianese continued, so machine learning could simply add to their toolkit.
When government uses machine learning merely as a decision aid, and not as a complete substitute for human decision-making, that too should make it relatively easy to defend, Coglianese continued.
In Allegheny County, the child risk prediction model aids, rather than replaces, the case worker’s decision to open an investigation.
All three panelists, Coglianese, Goldsmith, and Culhane, weighed in on the importance of community and social engagement as government agencies gradually adopt machine learning tools.
Part of Allegheny County’s success, Culhane explained, hinged on stakeholder outreach during planning, testing, and monitoring the new child risk assessment algorithm. He reported that this outreach not merely assuaged ethical concerns but transformed citizens into supporters of the program. When the model improved risk detection so significantly, the “ethical conversation flipped” and citizens started to think that it “would be unethical not to use the computer model,” Culhane said.
Looking ahead, the presenters predicted that machine learning adoption by state and local governments would continue to gain momentum. County officials from Philadelphia and across the country have already visited Allegheny County to learn about its child risk assessment model, so other jurisdictions may soon follow suit.
In developing and implementing the Allegheny Family Screening Tool, the Allegheny County Department of Human Services worked with an international research team led by Rhema Vaithianathan, a professor of economics at Auckland University of Technology in New Zealand, and which also includes Emily Putnam-Hornstein, an associate professor at the University of Southern California. Culhane works with Allegheny County through the Actionable Intelligence for Social Policy Initiative at the University of Pennsylvania, but he had no role in creating or implementing the county’s risk screening tool.
This workshop was the final one in a series of events making up the Optimizing Government Project, sponsored by the Fels Policy Research Initiative and the Penn Program on Regulation.
This essay is part of a seven-part series, entitled Optimizing Government.