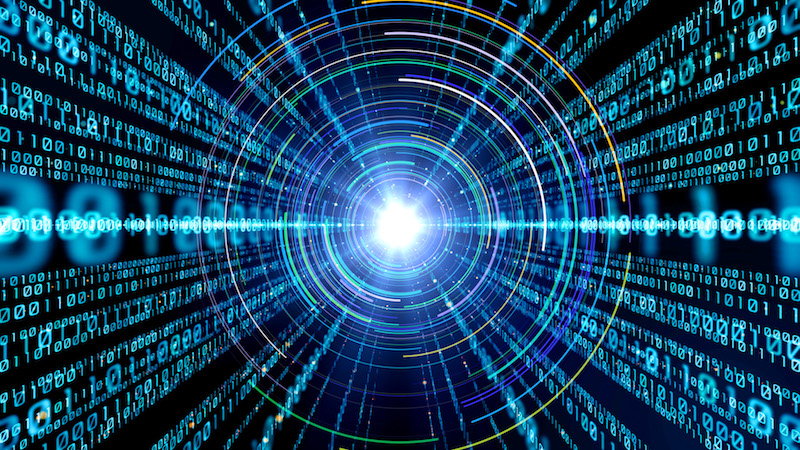
Randomized policy research should be pre-registered to reduce the chance of false positive results.
Randomization is a key ingredient of rigorous causal inference. Given the increasing desire for rigorous evaluation of the effectiveness of government programs, there are excellent reasons to start designing public policies with randomized implementation plans from the outset. But convincing government agencies to randomize policies does not necessarily ensure that researchers will reach consensus about their effectiveness. After all, randomization is not the only ingredient of good causal inference.
A major concern when randomizing public policy implementation is the risk of multiple hypothesis testing. If not properly accounted for, this problem could result in expensive, controversial efforts that produce little more than a morass of inconclusive results.
Simply put, multiple hypothesis testing occurs when researchers use the same data to test many hypotheses simultaneously—or if not simultaneously, at least separately. The problem with doing this is that conventional tests for statistical significance are more likely to produce false positives when dozens of hypotheses are tested using the same data.
In statistical analysis, sometimes an observed difference between two experimental groups is due to random chance rather than the result of the treatment being evaluated. To guard against these false positives, experimental researchers typically require a p-value below a certain threshold before they declare that a treatment has a causal relationship with an outcome. For instance, with a p-value below 0.05, experimenters may say that an observed difference between two groups is likely not due to random chance—or, more accurately, that there is a 95 percent probability that the same result would not have occurred if the treatment had no effect.
The problem is that p-values of 0.05 or below are likely to occur randomly 1 in 20 times, or 5 percent of the time. That is a small likelihood in a single experiment, but if 20 different researchers evaluate 20 different aspects of a policy intervention, even if the intervention did nothing, we should expect one statistically significant result and 19 statistically insignificant results.
This sounds complicated, but it can be explained with a simple example. Imagine that a team of researchers decided to run an experiment on 2,000 law students. Next, imagine they used that opportunity to give 1,000 of those students a red-colored placebo pill every day for three months, and to give the 1,000 other students a chemically identical blue-colored placebo pill every day for three months. If the researchers then tested whether the red group had lower anxiety levels than the blue group, odds are they would be statistically indistinguishable. After all, both pills were placebos. But if the researchers also tested 19 other outcomes—such as whether there were differences in the two groups’ blood pressure, cholesterol, weight, and so forth—odds are that they would find one statistically significant difference between the groups even though the pills did not actually do anything.
This would not be so bad if the results for all 20 outcomes saw the light of day, because we could just figure out afterwards that we should downgrade our confidence that the single statistically significant result is genuine. But due to the publication bias favoring experiments that find significant outcomes, odds are good that the single statistically significant outcome would be published and that many of the 19 outcomes where no difference was found would be left in the proverbial file drawer.
This problem is hard to solve for academics when they design research projects, but it is even harder when government agencies design public policies that produce publicly available data.
Even if the government decides to randomize the implementation of a policy, many agencies may not be interested in designing or carrying out their own evaluation of that policy. Instead they will outsource it to independent researchers who may test the data in dozens of different ways, increasing the likelihood of a false positive result.
And even if agencies do their own program evaluation, they cannot stop teams of enterprising social scientists from undertaking their own analyses of the publicly available data and creating the same probability problem.
The result is that the government may randomize the implementation of a public policy, but the results published about the policy’s effect may be incomplete or lack critical context. Without knowing all the hypotheses that were separately tested, it will be difficult to know what to make of the randomized policy overall.
This concern is much less problematic when private firms run their own consumer testing. A company like Google can randomize a customer experience and credibly commit beforehand to looking at only certain outcomes. This ensures that there will not be excessive multiple hypothesis testing. But when a government agency does the same thing, it is tough to prevent the public from getting the data and cutting it dozens of different ways.
The natural solution is to push government agencies not only to randomize their implementation of policies, but also to follow the current best practice of pre-registering their research designs. Pre-registered research designs commit the experimenter to conduct only predetermined analyses, which helps ensure there will not be dozens of subsequent ex-post analyses leading to spurious results due to random chance. It is still possible that a result produced by a pre-registered plan is due to random chance, but it is certainly less likely to be a problem.
It is a good sign that academics are pushing for randomization and evaluation of public policies, but we should also push for best practices, like pre-registration, across the board. This will help ensure that any policy experiments that the government conducts do not produce inconclusive results.
This essay is part of a 13-part series, entitled Using Rigorous Policy Pilots to Improve Governance.