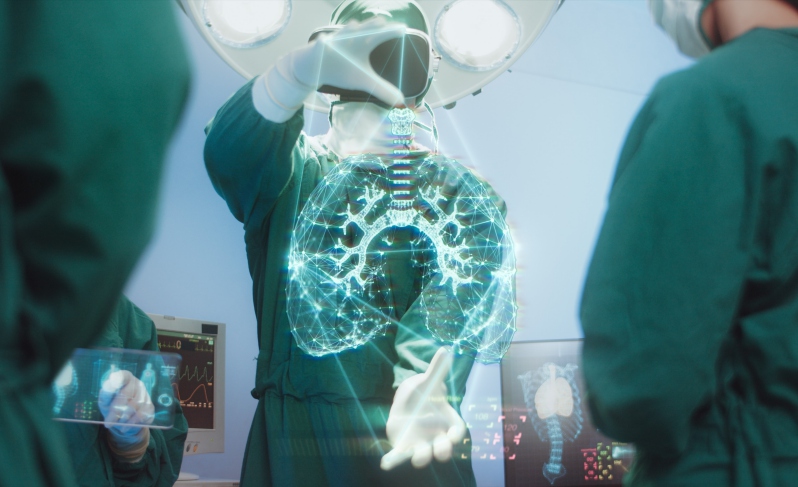
In regulating AI in medicine, FDA should exercise care in implementing a principles-based framework.
Artificial intelligence (AI) has the potential to make health care more effective and efficient in the United States and beyond—if it is developed responsibly and regulated appropriately. The Food and Drug Administration (FDA), which reviews most medical AI, only approves AI in a “locked” or “frozen” form, meaning it can no longer learn and adapt as it interacts with patients and providers. This important safeguard ensures that AI does not become less safe or effective over time but also limits some of its potential benefits.
To promote the benefits while still managing the risks of AI, FDA has proposed a new program to oversee “unlocked” AI products that can learn and change over time.
FDA’s proposal calls for a complex and collaborative approach to regulation that looks quite similar to the way financial regulators in the United Kingdom operated before the global financial crisis of 2007 to 2008. Financial regulators in the U.K. used a principles-based framework, which establishes broader principles in lieu of more detailed rules.
This style of regulation failed during the financial crisis, but that does not mean FDA’s AI proposal is doomed. It does, however, mean that regulators and Congress should take a few lessons from where things went wrong in the financial crash—especially by ensuring regulators can stay independent from the companies they oversee and by placing equity at the very heart of the regulatory equation.
Taking a step back, AI promises advances in several fields of medicine. For example, providers can use AI to make diagnoses for patients by looking at their medical imaging or even pictures of their skin. Recent evidence suggests AI can be much better at making accurate diagnoses than even trained radiologists or dermatologists, so using software could cut costs and delays for getting a diagnosis in a routine case.
Despite its potential, the risks of medical AI still need to be managed. Unfortunately, it is not always easy to determine how an AI system makes a diagnosis or medical recommendation because AI often cannot explain its decision-making.
AI software does not “know” what a human body or disease is, but instead, it recognizes patterns in the images, words, or numbers it “sees.” This limitation can raise real questions about whether an AI system is making the correct diagnosis or recommendation and how it arrived at that conclusion.
Big problems can result from AI systems that analyze data with bias. The U.S. medical system has a long history of racism and other forms of marginalization, which can be reflected in medical data. When AI systems learn using biased data, they can contribute to worse health outcomes for marginalized patient groups. Regulation to prevent these inequitable outcomes is critical.
Many applications of medical AI would fall under FDA’s authority to regulate medical devices. The 21st Century Cures Act does exclude some types of “low risk” AI from FDA review, such as algorithms designed to assist physicians. But many AI products still must go through FDA review—putting the agency in a position to take their risks seriously.
In April 2019, FDA released a white paper describing an innovative regulatory plan for unlocked AI software. FDA’s AI proposal builds off of its previous idea of “pre-certification,” which would have the agency regulate developers as a whole, instead of their individual software products, using broad principles such as “clinical responsibility” and “proactive culture.”
The 2019 AI proposal adds to this framework by asking developers to describe how they expect their software to change over time and how they will manage risks from those changes. FDA, with help from developers, would then watch for real-world outcomes in clinics and might require further regulatory review if the software changes too much.
The net effect is a regulatory system in which FDA approves a software developer’s plans for self-regulation in AI development, then uses the pre-certification principles to evaluate regulatory outcomes and determine if they align with public policy goals. This type of system could be called principles-based regulation, which is the approach that British financial regulators used prior to the global financial crisis and others continue to use today.
Earlier in 2021, FDA announced plans for advancing the proposal and responding to stakeholder comments. Should FDA continue to move forward with its proposed principles-based plan, there are important lessons to take from the global financial crisis.
First, although regulators can learn and adapt to new and complicated situations or technologies by working with the companies they oversee, regulators still need to maintain independence from those companies.
A core part of this lesson requires regulators to have a big enough budget to oversee the industry effectively. Regulators need enough resources to supervise companies and develop their own internal expertise about the technologies and markets they regulate, so they do not rely too heavily on companies for expertise and expose themselves to the risks of capture.
Second, regulatory failures can often harm marginalized groups the most, which the financial crash showed yet again. Already, peer-reviewed reports have shown incidents of algorithmic bias leading to worse medical care for Black patients—meaning AI could be more or less safe and effective for different patient groups. Failure to regulate this component of AI could lead to unacceptable, inequitable health harms.
To address these issues, policymakers should consider at least two actions. FDA should ask Congress for a long-term budget boost when the agency requests new legislation to implement the AI plan—and Congress needs to be willing to fulfill the agency’s request. Furthermore, “health equity” should be used as a standalone principle or outcome by which the agency will measure company performance and real-world outcomes. These and other modifications to FDA’s AI proposal could set regulators up for greater success in protecting all patients.
Congress and civil society groups should also monitor this complex area of policy and regulation to make sure that AI in medicine does make society healthier, safer, and more equitable.