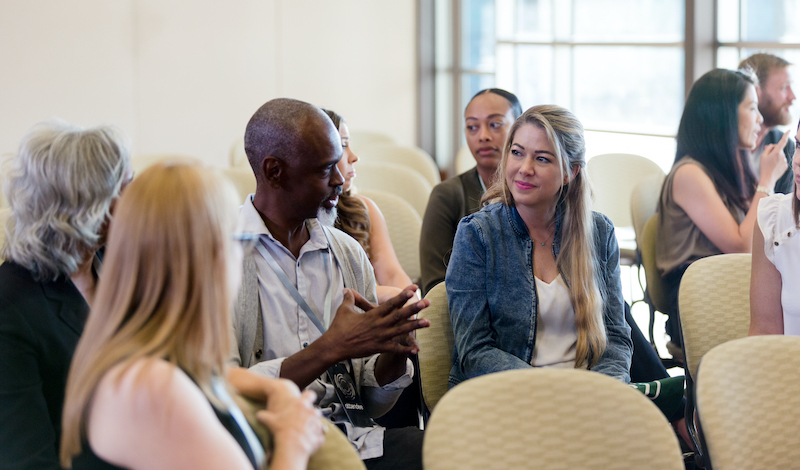
Using artificial intelligence in public consultation processes could enhance participation in rulemaking.
Consultation with the public is widely recognized as a tool to ensure participation in rulemaking while enhancing legitimacy and trust in regulatory agencies.
Many experts have already discussed how to enhance stakeholders’ participation in consultation, an outcome that does not automatically follow by formally opening consultations to everyone. At the same time, how to give a voice to marginalized groups—typically, citizens and small businesses—is still open to debate, both in Europe and the United States. Well-recognized approaches to deal with the latter issue include using e-consultations, multiple consultation methods, and tailored consultation documents.
But recently, a new question has spurred scholarly debate: Should regulatory agencies use computational tools to enhance participation in consultations? Indeed, some government officials already employ artificial intelligence (AI) to process and analyze comments in consultations.
The European Commission shows a mixed attitude in this regard. On the one hand, it uses text processing technologies such as Atlas.ti to analyze comments collected in consultations with large numbers of participants. On the other, the Commission’s Better Regulation Toolbox 2021 includes recommendations for only using data analysis software, such as STATA or NVio, for identifying the existence of organized interests.
Computational tools can help agencies solve problems presented by mass comments, as hinted by the Administrative Conference of the United States (ACUS). For example, technology can de-duplicate identical comments. In addition, automatically computer-generated comments may need to be flagged and stored separately from other comments, which regulatory agencies can accomplish with AI to save considerable time and resources. At the same time, however, these systems may sometimes automatically exclude mass comments or consider them as a single comment, which could misrepresent the level of public support behind the comments.
AI tools can also increase public participation in consultation through social media. ACUS recommends that regulatory agencies take special care when using social media platforms to gather lay comments. Even though citizen narratives add detail to the regulatory discourse, they are hard to assimilate to the managerial, rational language used by decision-makers, and they seldom translate to input to the rulemaking process. According to the European Court of Auditors, this outcome can generate feelings of alienation in citizens, who fear being ignored by rulemakers.
Against this background, there is another important and underexplored field where computational tools could enhance participation: identification of interest groups.
Language-based computational tools can help identify interest groups and give voice to marginalized groups. Members of these groups participate in consultations through documents and narratives that are digitized, which can then be analyzed with tools such as natural language processing (NLP), which allows computers to recognize and analyze speech.
In a recent paper, my co-authors and I demonstrated the feasibility of using NLP techniques to help create clusters of stakeholder groups in consultation processes based on semantics—that is, the way that groups understand and use key words and expressions related to a given policy. We term this process “language-based stakeholders clustering” (LBSC).
LBSC can use linguistic tools in different ways to identify stakeholders. For example, word embedding is a tool that computes the semantic value of different words in a text and creates groups based on people’s shared understanding of the meaning of words. Topic modeling is another technique that scans the frequency and pattern of words in a text and creates groups based on shared topics. A further technique, sentiment analysis, can parse text and then create groups based on people’s shared opinions, preferences, and feelings about a topic.
Linguistic analysis of people’s replies to consultations offers several advantages. Not only does this new methodology increase the empirical evidence that rulemakers have at their disposal, but it may also help overcome barriers to participation, especially for citizens and small businesses. LBSC could analyze narratives from different sources, whether newer sources such as social media posts or classic sources such as replies to questionnaires or feedback documents.
Interestingly, LBSC may lead to clustering stakeholders in ways that are different from clusters that result from traditional qualitative and quantitative analyses. This outcome can occur—as it did in our work—because LBSC gathers insights from stakeholders’ own written language.
For instance, based on individuals’ replies to the consultations over what later became the Digital Services Act and Digital Markets Act proposals, LBSC clustered three groups: individual/micro organizations, small organizations, and medium-large organizations. These clusters were justified by statistically significant differences in the way that stakeholder groups used and understood key terms, such as “gatekeepers,” “self-regulation,” and “readability.”
Using LBSC, we ascertained that even groups belonging to the same community often understand key terms very differently, suggesting that not all groups voice the same concerns even when they say the same words. We could also quantify the difference between each stakeholder groups’ positions in the consultation. This finding could aid further research, as it may help to clarify which positions expressed during consultations later translated into actual rules.
Computational tools, if combined with traditional ones, may provide a more robust understanding of the language used by marginalized groups and what they want. Also, clustering groups based on linguistics can help further participation of the least organized groups, by assigning them true “voice” and providing incentives for them to participate. Consequently, rulemakers should consider using these techniques in combination with traditional tools to enhance participation and effectiveness of consultations.