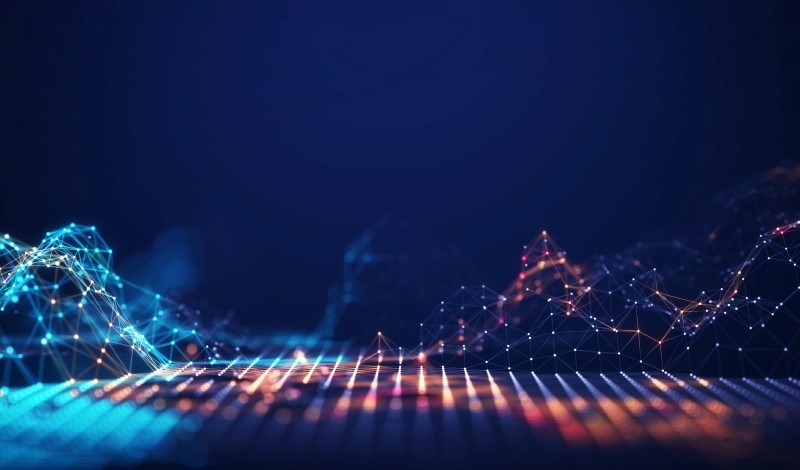
Antitrust regulators can improve their performance by relying on responsible use of artificial intelligence tools.
As technology changes, markets change too. Recent releases of tools based on large language models, such as ChatGPT and Bard, are now supporting a broad range of new digital applications that appear likely to bring significant disruptions—positive and negative—to aspects of the economy and society at large.
At the same time that the private sector adopts new uses for artificial intelligence (AI), these algorithmic innovations place new demands on government regulators. They also present regulators with new opportunities. The same digital tools that drive innovations in the private sector can—and in some cases must—be deployed to improve regulators’ ability to oversee markets. Traditional approaches to regulation that rely merely on adopting new rules or hiring more audit staff will no longer be sufficient to oversee technologically complex and increasingly networked markets.
Like other regulators, antitrust regulators face similar demands and opportunities created by new algorithmic tools. Competition authorities have always faced far more transactions and firms than they could ever possibly monitor at close range. But today, machine-learning algorithms can help regulators better identify suspicious activity and allocate scarce oversight resources.
Algorithmic antitrust regulation might also be needed to confront a growing reliance on dynamic pricing algorithms in private markets that, while potentially leading to consumer welfare gains, can also open up new possibilities for anti-competitive behavior. Algorithmic price-setting has the potential to create both intentional and unintentional market distortions, making it difficult for regulators to distinguish between legitimate market efficiencies and anti-competitive practices. With autonomously learning algorithms, market collusion may even occur without any human input, further complicating the regulator’s task.
To address these challenges, antitrust authorities may well need to modify certain antirust rules. But at a minimum, they must adapt to the changing marketplace by pursuing when appropriate the use of similar algorithmic tools as part of their oversight efforts. The increasing complexity of business behavior and reliance on sophisticated digital technology make it crucial for regulators to consider how they can make the best of these advancements too.
Other regulators are already using big data and machine-learning algorithms to maximize the impact of their limited enforcement resources. The U.S. Internal Revenue Service uses AI tools to help in detecting tax evasion, while the U.S. Securities and Exchange Commission uses these tools to detect securities fraud. One study has shown that environmental regulators could use machine-learning algorithms to improve their detection of violators of water pollution rules by 600 percent.
Antitrust authorities have so far tended to be slower to adopt AI tools, but interest is starting to grow in the face of calls for so-called computational antitrust. Competition regulators are increasingly taking an interest in possible ways these technologies might supplement or enhance traditional antitrust tools. Lawyers and economists are also recognizing the potential of AI tools to process vast quantities of data and more effectively analyze conditions of market competitiveness.
Transitioning to an era of “antitrust by algorithm” will not be easy. Antitrust regulators will need to make decisions about when and for what purposes to use what specific kinds of algorithmic tools and how those tools should be designed and deployed.
In making these decisions, regulators may benefit from a report I wrote for the Administrative Conference of the United States in which I provided a framework for governmental decision-making about the deployment of AI tools. As I noted in that report, regulators do not need to determine that AI tools will work perfectly before they choose to use them. They do, though, need assurance that AI tools will outperform the status quo which has largely relied on human decision-makers, with all their foibles.
Deciding whether to rely on AI tools to perform regulatory audit and oversight functions should take into consideration several basic preconditions for the viable use of AI. The regulator’s goals for any AI tool must be capable of being articulated in a mathematically precise manner. Sufficiently large quantities of usable data must also be available. And those data should be current and suitably related to the desired goal.
Data availability can be a challenge, as firms will often be reluctant to share their data with regulators. Data-sharing requirements may need to be expanded. New opportunities for real-time data sharing may arise if businesses increase their use of blockchain technology.
Data sources, it is often said, have become akin to the new energy sources fueling the economy—and regulators will need to find ways to capture relevant data and organize and store it in useable form. For this purpose, antitrust regulators will need adequate hardware, cloud computing capacity, and well-designed internal procedures to combat cybersecurity risks.
Like other regulators, antitrust regulators will need to audit and validate the AI tools they develop and deploy. The goal with these tools should be to improve on the status quo.
Fortunately, with the data needed to use AI tools in the first place, regulators should be able to monitor and test their tools to ensure they are making a positive difference and not creating undue harms. Regulators should be mindful, though, of the possibility of untoward biases that might be captured or reinforced by algorithmic tools. Antitrust authorities must be prepared to identify and mitigate any biases propagated by any machine-learning algorithms they use.
Like other organizations, antitrust regulators will benefit from robust internal self-auditing and AI risk management practices. These would likely include data privacy and security procedures, staff training, regular reporting protocols (perhaps via model cards), and independent oversight.
Making the most of advances in AI tools will, perhaps counterintuitively, demand significant attention to building human capital within antitrust agencies. Even when government agencies rely on outside contractors to use AI tools or build automated systems, they will need some degree of in-house expertise in data analysis, not to mention adequate human resources to oversee the legal and ethical issues related to artificial intelligence use. Building and maintaining adequate in-house expertise will not come easily, given the higher pay offered in the private sector for analysts with similar skills.
Despite these challenges, a future of antitrust by algorithm has great potential to improve antitrust regulation and enforcement. Machine-learning tools promise antitrust authorities the possibility of better identifying and addressing anticompetitive behavior, which can lead to a more competitive marketplace and better outcomes for consumers. And by taking well-considered steps in deciding when and how to use artificial intelligence, antitrust authorities can help ensure that antitrust by algorithm is carried out in a fair and responsible manner.