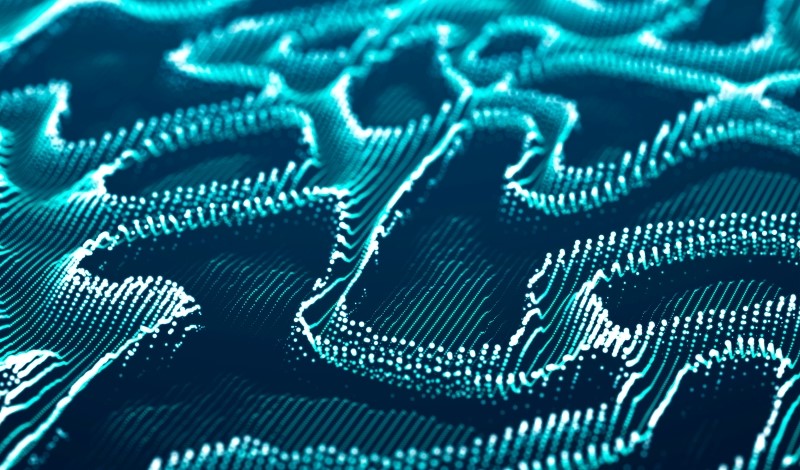
Artificial intelligence can help agencies review and improve their existing regulations.
Statutes, executive orders, and good governance considerations alike impose a duty on many federal agencies to analyze past rules periodically and to remove duplicative, inconsistent, anachronistic, or otherwise ineffective regulations from their books. To comply, agencies perform “retrospective reviews” by re-assessing the costs and benefits of their regulations at some time after the regulations are issued.
This longstanding practice of retrospective review has been endorsed in various Administrative Conference of the United States (ACUS) recommendations since 1995.
Executive orders from the Obama, Trump, and Biden Administrations expanded many agencies’ retrospective review obligations. More recently, agencies have begun to consider how technology might maximize the scope of the review they can perform, notwithstanding limited resources. For example, the Office of Management and Budget suggested in its November 2020 guidance that artificial intelligence (AI) might be an effective tool “to promote retrospective analysis of rules that may be outmoded, ineffective, insufficient, or excessively burdensome” and to “modify, streamline, expand, or repeal them in accordance with what has been learned.”
Observing this trend toward algorithmic review, one of us (Sharkey) proposed a study to ACUS and produced a report assessing how certain agencies now use, and how others plan to use, AI technologies in retrospective review. Prior to the ACUS study, little was known about agencies’ use of algorithms to facilitate retrospective review, so Professor Sharkey first endeavored to conduct field studies to discover relevant uses of AI-enabled technology.
Four representative case studies proved instructive: three from cabinet-level executive branch departments—the U.S. Department of Health and Human Services (HHS), the U.S. Department of Transportation, and the U.S. Department of Defense—and one of a project by the General Services Administration (GSA) in collaboration with the Centers for Medicare and Medicaid Services (CMS).
The first case study on HHS’s use of AI technology was particularly striking. The department christened its 2019 AI pilot project “AI for Deregulation,” a clear statement of its intent to use AI to remove rules rather than repair or replace them. In November 2020, HHS launched a “Regulatory Clean Up Initiative” through which the department has applied an AI and natural language processing (NLP) tool created by a private contractor to HHS regulations. This culminated in a final rule that corrected nearly 100 citations, deleted erroneous terms, and rectified many misspellings and typographical errors. The public only learned of the AI tool and its use by HHS upon the issuance of the rule.
The second case study explored retrospective review at the Transportation Department, which reviews all of its regulations on a ten-year cycle. To date, the Transportation Department’s largest AI-based retrospective review tool is its “RegData Dashboard,” which, according to internal documents, seeks to “apply a data-driven approach to analyzing regulations” to “inform policy decisions, analyze trends, provide management reports/monitoring, and display the entire ‘lifecycle’ of regulatory actions.” The Transportation Department’s Office of the Chief Information Officer built the RegData Dashboard to implement QuantGov—an open-source policy analytics platform developed by the Mercatus Center—which uses data tools to analyze regulations and estimate their regulatory load.
The Transportation Department’s algorithmic tools exert a unique influence on the agency’s rulemaking process. The department drafts its rules to fit a structured, agency-wide format designed to organize key meta-data elements, such as who or what the regulated entity is and who is responsible for enforcement. Compared to a less structured approach to drafting rules, the Transportation Department’s consistent format makes it relatively straightforward for subject-matter experts to encode the substance of Transportation Department rules into a “machine-readable” format, thus decreasing the cost of “teaching” the Transportation Department’s algorithmic tools the semantic meaning of regulatory text and obviating the need for NLP.
The Defense Department presents a third example of an agency’s use of algorithmic tools to perform retrospective review. The Defense Department faced a thorny problem: a “mountain of policies and requirements” authored by various officials and officers across its constituent agencies and military services. The Defense Department’s rules constituted a sprawling, decentralized structure which rendered many forms of coordination prohibitively difficult. In response, Congress required the Defense Department to create a “framework and supporting processes” to ensure retrospectively that the department’s shared intelligence community “missions, roles, and functions” are “appropriately balanced and resourced.”
Thus, the Department created “GAMECHANGER,” an AI and NLP tool which centralized a structured, unified repository for its entire catalogue of guidance. GAMECHANGER also assists the Defense Department staff in drafting new policy documents to avoid conflicting with or duplicating prior department positions. The Defense Department prototyped GAMECHANGER in-house before transferring development duties to a contractor tasked with supporting other Defense Department data analytics tools. According to one Defense Department official interviewed, GAMECHANGER decreased the time of responding to policy-related queries “from months to seconds,” and saved almost $11 million in annual costs.
The final case study explored a GSA initiative to leverage its centralized technical expertise to provide shared software for government. GSA partnered with CMS—an executive agency within HHS—to perform a pilot study into “cross-domain analysis,” which the agencies hoped would reduce burdens and avoid duplicative regulation by coordinating rules across regulatory domains.
Since the Dutch government had implemented similar technology in its health care and immigration services, GSA and CMS sourced the technology for the project from two Dutch organizations. The ensuing three-month study investigated a test case of CMS regulations governing subsidies for portable oxygen systems. CMS had already identified inconsistencies in the selected rules, and the Dutch companies’ tools were able to locate the expected contradictions successfully.
In addition to performing these case studies, Professor Sharkey interviewed officials from eight other agencies about their interest in using algorithmic tools for retrospective review. These interviews revealed that, for the most part, agencies’ retrospective review methods are nowhere near automated. The U.S. Department of Education, for example, performs retrospective review by a completely manual process which one official described as “pretty labor intensive.” Agency representatives theorized that concerns with the government’s capacity and resources to develop AI tools for retrospective review had stymied adoption, and none thought it would be realistic to develop such tools in-house.
Nonetheless, officials at all but one agency were open to the use of AI-enabled tools in the retrospective review process, and several interviewees pointed out particular agency tasks that might lend themselves to automation, such as finding broken citation links or flagging long-standing, unedited regulations as ripe for review.
To obtain another perspective, Professor Sharkey surveyed regulatory beneficiaries and regulated entities using a sample list provided by ACUS. Of the six regulatory beneficiaries and one regulated entity that agreed to be interviewed, most said their chief concerns with AI-enabled tools for retrospective review were AI trustworthiness and explainability. Indeed, two self-described “AI skeptics” contended that much regulatory text is too context-specific and difficult to unpack for an algorithm ever to replicate or replace an agency’s expertise and experience. The other interviewees, however, were at least cautiously—and some, enthusiastically—supportive of exploring the use of AI in reviewing regulations. One interviewee said that “AI is key in retrospective review, because no one wants to do the work, and it’s low priority; so AI is perfect for that.”
The report and case studies are, we think, both encouraging and instructive for governmental creators and users of AI. One lesson learned is that the resources and technical expertise required to carry an AI project to the finish line are rare among federal agencies. Where internal capacity exists, agencies should consider launching pilot projects on algorithmic retrospective review and sharing their tools openly with other federal agencies. The Defense Department’s AI tool, GAMECHANGER, for example, sparked interest in creating spinoff projects across the government, both at individual agencies, such as the U.S. Patent and Trademark Office, and at agencies with a cross-government focus, such as the Office of Management and Budget.
Another position we take, more uncompromisingly, is that AI tools for retrospective review must be open source and able to operate in synergy with other government technology initiatives. GAMECHANGER again is a shining example: nearly all its code is available on open source platforms, so any agency interested in implementing it would incur relatively low startup costs. Likewise, GSA required that its pilot with CMS be open source and compatible with common government-used data architectures such as United States Legislative Markup. By choosing to prioritize interoperability and to stay open source, the agencies created non-proprietary, widely shared tools and developed internal technical capacity to insulate themselves against the possibility of being locked into contracts with a single vendor.
Finally, we suggest that the case studies of agency experience with AI in retrospective review also shed light on the value of AI at the moment of prospective rulemaking. An example of how AI-enabled retrospective review could come into play at the time of rulemaking is the Transportation Department’s practice of drafting regulations in a structured format which facilitates better comprehension of rules by computers. Algorithmic retrospective review tools could also be used throughout the lifecycle of crafting new regulations to ensure that the new rules are well-drafted, consistent, and non-duplicative.
It may well be that, in the near future, AI will wear many hats in the rulemaking process, such as modeling the effects of policy choices or gauging their costs and benefits. Easing AI into prospective rulemaking by learning from and replicating its contributions to retrospective review is, in our view, a prudent first step.
This essay is one of a four-part series on Using Technology to Improve Administration.