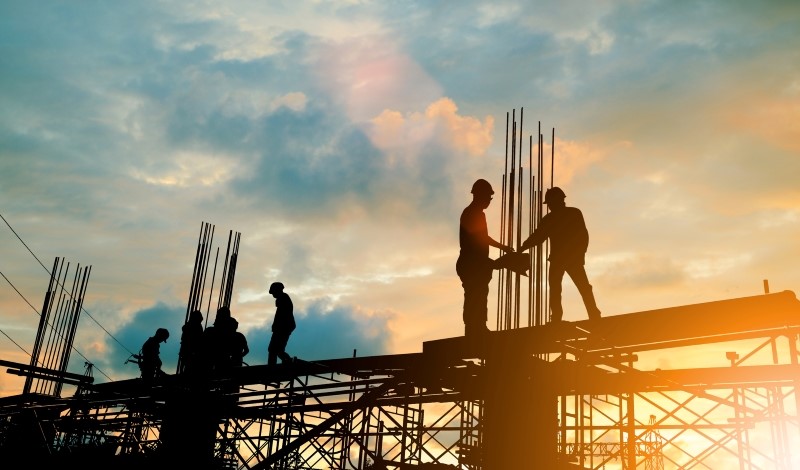
OSHA should use machine learning to improve the effectiveness of its regulatory inspections.
Government agencies charged with improving worker safety, tax compliance, environmental protection, and other concerns routinely face difficult decisions about how to inspect the workplaces they are responsible for monitoring. This is a serious challenge given agencies’ acute resource constraints that allow them to inspect only a tiny proportion of those sites. And with dysfunction on display in the U.S. Congress’s recent attempts to pass a budget, federal agencies’ resource constraints are unlikely to improve anytime soon.
Under these circumstances, our new research shows that regulatory agencies can substantially benefit by using machine-learning tools to target their limited inspection resources.
Take the U.S. Occupational Safety and Health Administration’s (OSHA) enforcement budget, for example, which funds its mission to “assure safe and healthful working conditions” in over half the states across America (the other states operate their own agencies, overseen by OSHA). OSHA and its state partners inspect less than 1 percent of the millions of workplaces they oversee. Despite decades of concerns that OSHA has insufficient inspectors, a recent Republican proposal suggested cutting the OSHA budget by 15 percent. With such limited resources, how can agencies such as OSHA use their inspections to achieve their mission—and use taxpayer dollars wisely?
Our study examined tens of thousands of workplaces across 28 states and found that OSHA inspections reduced injuries by 9 percent, broadening the implications of our earlier study published a decade ago that found California workplaces experienced a 9.4 percent decline in injury rates and a 26 percent reduction in injury costs after safety inspectors randomly targeted them. Combined, these studies demonstrate that the safety-improving benefits of regulatory inspections are widespread and not limited to a single state.
But perhaps more importantly, our recent study also found that OSHA could prevent as much as twice as many injuries—translating to up to 16,000 fewer workers injured and nearly $1 billion in social benefits over the decade we examined—if inspections were targeted based on alternative approaches that we designed and evaluated.
How can such a tremendous gain in regulatory effectiveness occur? OSHA, like many other regulatory agencies, tends to prioritize inspections for workplaces with prior records of high injury rates. Those workplaces, however, might not be where inspections can do the most good.
Targeting workplaces based on their prior records is no guarantee that those are the workplaces facing the most hazardous conditions today. Moreover, if the regulator’s goal is to improve safety, others have pointed out that OSHA should in theory focus on where inspections can prompt the biggest reduction in injuries, which are not necessarily the workplaces that have the worst injury records. Fortunately, recent advances in machine learning tools enable agencies to overcome both of these shortcomings.
We find that, without changing its inspection budget, OSHA could have prevented twice as many injuries if it instead used machine learning prediction algorithms to target inspections toward workplaces predicted to be particularly responsive to inspections—those that would do the work to actually reduce injuries following inspections. Moreover, we find similar gains if OSHA were to target workplaces predicted to have the most injuries at the moment when inspectors arrive, and not merely in the past.
This potential for improved effectiveness is by no means limited to OSHA and workplace safety. Other recent studies have shown how machine learning can improve inspections’ effectiveness in areas ranging from restaurant hygiene to water pollution.
Of course, these gains must be balanced against concerns about transparency and fairness that can arise given the “black box” nature common to many machine learning prediction algorithms. These are important discussions to have. But with regulatory budgets under pressure in many countries, states, and municipalities, it is more important than ever for government agencies to make the most effective use of their resources. Our study demonstrates how machine learning offers a way to do just that.