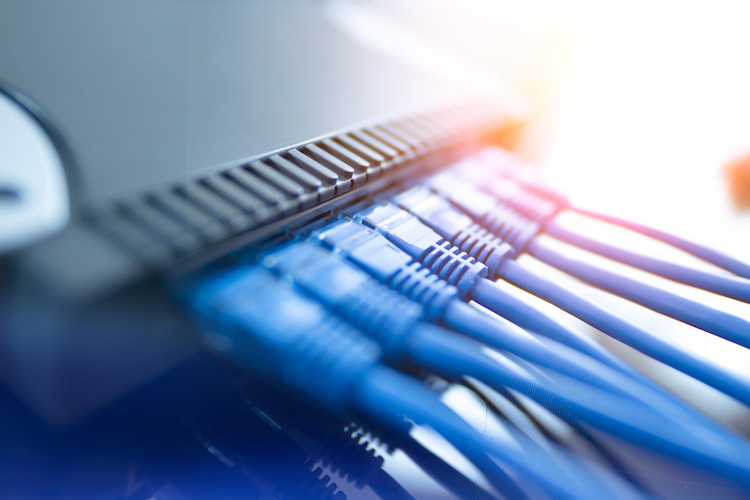
Misaligned incentives can encourage incomprehensibility.
The precept “of the people, by the people, for the people” demonstrates not only that citizens must choose leaders through accountability processes and institutions, but also that each citizen’s decision-making must be as informed as possible. This vision can only be accomplished through the availability of valid and relevant information that must be understandable to all audiences.
Over the last two decades, policy experts have hoped that novel technologies would be used to make information more meaningful, but many of these expectations are still unfulfilled. In her book with Will Walker, Incomprehensible!, Wendy Wagner demonstrates that various legal programs are built on the foundational assumption that more information is better, ignoring the imperative of usable and meaningful communication.
The design of many legal programs favors the production or reporting of undigested information, which is in turn passed along to an unequipped, disadvantaged audience. Wagner argues that although there are numerous procedural steps required for Congress to pass laws, there are no institutional controls that require a bill to be comprehensible to other members of Congress. This suggests that even today there remains an endemic, fundamental problem of unintelligibility.
The principle of governmental transparency is only fulfilled when information is relevant and understandable to a general audience. Unintelligible information or the mere release of unprocessed data does not fulfill the principle of transparency. On the contrary, it opens the doors wide for parties with technical expertise to profit from their strategic advantages over the less empowered. This concern is particularly relevant in the face of modern challenges, such as misinformation and the lack of actors that process information on behalf of citizens.
Automating government processes through machine learning would have uncertain implications in this regard, especially when the inner workings of those processes are unintelligible and might not benefit the average citizen, as Wagner argues.
Scholars have argued that machine learning can meet the law’s demands for transparency and does not contravene the principles of nondelegation doctrine, due process, equal protection, and reason giving. It also can enhance efficacy, efficiency, and legitimacy in government. Principles of fair algorithmic governance, however, go beyond mere disclosure and understandability of the technical aspects of machine learning resources, such as the source code, data, objective function, parameters, and training data sets. Algorithmic governance is rooted in the very ecosystem over which those technical resources are applied and operate.
Thus, even if these technical resources are put into the open, they will introduce even more confusion if they are applied to a convoluted law that can only be understood by selected parties—with a narrow exception for machine learning that is applied to make laws more meaningful to a wider audience. Applying algorithm-based decisions to an ecosystem of unintelligible laws or regulations that favor a few knowledgeable stakeholders will compound any endemic problem, particularly if these very stakeholders further their agenda through their knowledge of machine learning. This situation would worsen the already fragile ecosystem to which Wagner refers.
The future of machine learning in government is therefore uncertain, because the technology is applied where processing help is needed, but also where it is convenient for stakeholders with great knowledge and agendas that might not be aligned with the average citizen.
According to Cary Coglianese, algorithms will likely be applied more often to assist, rather than to supplant human judgment. Indeed, the judiciary in the United States has been cautious and rather slow to utilize algorithms, mainly applying them to areas of risk assessment and dispute resolution. The majority of these tools are based on statistical approaches or conventional supervised learning logistic regression models, rather than unsupervised learning models.
Administrative agencies, on the other hand, seem to be way ahead of the judiciary. They have already employed full-fledged machine learning tools for various regulatory tasks—such as identifying cases of possible fraud or regulatory violations, forecasting the likelihood that certain chemicals are toxic, identifying people by facial-recognition when they arrive in the United States, prioritizing locations for police patrols, and more. As in the criminal justice system, none of this artificial intelligence has fully replaced human decision-making, with the exception of processes like the automation and optimization of traffic light and congestion avoidance, which has relegated humans to a “supervisory control” role, common of the automatic control field.
The application of machine learning to a government process is one of the last stages of a continuum in which algorithms become increasingly complex. This continuum starts with the processing of data which can offer meaningful visualizations, proceeds with the utilization of statistical approaches that can provide even more insights, and continues with the utilization of full-fledged machine learning approaches. The use of machine learning in governmental settings has not escaped controversy, particularly on the issues of bias, prejudice, and privacy that can arise from imperfect data. In addition to the fundamental issues Wagner addresses, various aspects of machine learning do not seem to be proper in early stages of this continuum, bringing a certain degree of pessimism about the application of machine learning in such an imperfect context.
My concerns are not unfounded. One example of the possible application of machine learning to an imperfect context is model legislation, also referred to as “model bills.” Unsuspecting lawmakers across the United States have been introducing these bills designed and written by private organizations with selfish agendas. For lawmakers, copying model legislation is an easy way to put their names on fully formed bills, while building relationships with lobbyists and other potential campaign donors. Model legislation gets copied in one state capitol after another, quietly advancing hidden agendas of powerful stakeholders. A study carried out by USA TODAY, The Arizona Republic, and The Center for Public Integrity found that more than 2,100 bills that became law in the last eight years had been almost entirely copied from model legislation.
Although the process of adopting model legislation—or algorithmic objects, as I call them, because they could be re-utilized—could be perfectly appropriate for bills with a proper purpose, the model bills passed into law often pursue the goals of powerful groups. Some of these bills introduced barriers for injured consumers to sue corporations, limited access to abortion, and restricted the rights of protesters, among others.
According to the study, model legislation disguises its authors’ true intent through deceptive titles and descriptions. The “Asbestos Transparency Act,” for example, did not help victims exposed to asbestos as its title implied; it was written by corporations who wanted to erect more obstacles for victims seeking compensation. The HOPE Act made it more difficult for people to get food stamps and was written by a conservative advocacy group. “In all, these copycat bills amount to the nation’s largest, unreported special-interest campaign, driving agendas in every statehouse and touching nearly every area of public policy,” note two reporters involved with the Center for Public Integrity in its recent study.
Open Government Data, a technical and policy stance favoring publicly available government data which will facilitate the upcoming adoption of machine learning, is another area of concern. Very expensive initiatives and data portals in the United States have raised expectations but have failed to change agency resistance to openness or invigorate public participation. On the contrary, these initiatives have created barriers to access by favoring individuals and organizations with highly technical skills.
The problem of unintelligibility is not limited to the United States. An assessment of international government portals indicates that data-oriented technologies are not being used to make things more understandable, signaling to the myopic work of influential international organizations that have pushed for expensive technical implementation while leaving aside the needs of disadvantaged audiences in spite of the explicit warnings a decade earlier.
These are a few challenges the regulatory community must address to be ready for the eventual application of machine learning. Wagner is right to highlight these challenges, and her book pinpoints suggestions for addressing them at a fundamental level.
This essay is part of a six-part series, entitled Creating Incentives for Regulatory Comprehensibility.