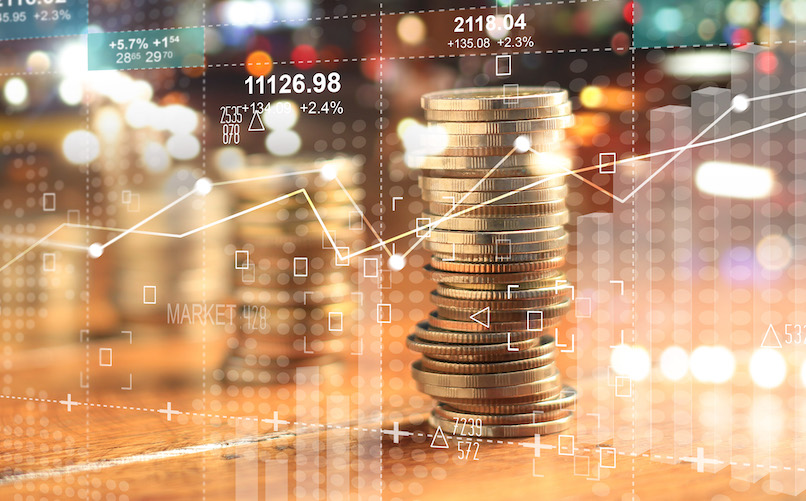
New quantitative analytics tools help economists understand links between regulation and income stratification.
Within the last three years, researchers in the nascent field of policy analytics have found links between areas of study which have traditionally been relegated to different corners of the economics profession. Using big data and machine learning techniques to leverage the power and storage capacity of modern computers, researchers are gaining a better understanding of the relationship between regulation and income distribution.
Economists have long understood that regulations have costly and unintended consequences, but generally have lacked the pertinent data to examine these theories empirically. Using data analytics and machine learning tools to test this relationship in greater depth, economists have discovered that regulations indeed increase income inequality.
Until the 1970s, economists’ perceptions of the government’s regulatory role were shaped by a theory formed during the darkest days of the Great Depression. Arthur Cecil Pigou’s public interest theory—which postulated that government regulation is required to protect the public from faulty products and correct market failures—held intellectual sway until the seminal work of George J. Stigler. Stigler found that special interest groups can be very effective at lobbying politicians and regulators for new rules that protect existing firms, thus stifling competition by discouraging new entry into an industry.
The phenomenon in which rulemaking is weaponized to protect incumbent firms—sometimes called capture theory—became the basis of the public choice theory of regulation. Although many regulations are designed with noble intent, some new legislation and regulatory law is inherently anticompetitive. Public choice theory predicts that a buildup of new regulation should correlate with a rise in economic frictions and reduced economic opportunities—especially among people and businesses who would otherwise be free to offer new or different services. This is how regulations, by creating distinct winners and losers, can increase income inequality.
Consistent with this theory, a 2003 study by William F. Shughart II and Robert Tollison reported that income inequality is significantly higher in states with more influential special interest groups that can impact regulatory outcomes in their respective states. This influence is perhaps best exemplified within labor market regulation. With respect to occupational licensure, researchers such as Morris Kleiner have shown that those who possess licenses to work in certain professions earn economic rents, widening the income gap between licensed and unlicensed workers.
It is no leap of faith to conclude that regulations, on balance and writ large, exacerbate inequality. Still, proving the connection remained elusive until the development of RegData, a database of industry-specific regulations developed by the Mercatus Center at George Mason University.
RegData’s algorithm counts each specific binding legal restriction—for instance, words implying an obligation such as “shall” or “must”—that appears in the Code of Federal Regulations. These restrictions are weighted by their industry relevance through a machine learning algorithm and summed to produce regulatory index values—numeric ranking or weighting based on the level of regulation—by industry and by year. This panel structure enables researchers to monitor and analyze regulatory restrictions within a particular industry over time. Using these new data, researchers have examined the multifaceted impact of regulations on the U.S. economy, including the widening distribution of income.
A 2019 study by James Bailey, Diana W. Thomas, and Joseph Anderson and another study conducted in the same year by Sean Mulholland used RegData to determine that higher levels of industry regulation are associated with wage inequality within occupations across different industries—for example, accountants working in different sectors—and between different occupations within a given industry—for example, production workers versus compliance workers working within the same sector. Bailey, Thomas, and Anderson found that greater industry regulation is associated with an increased disparity between high- and low-income occupations. Mulholland found that more than 40 percent of wage inequality within a given occupation is explained by variation in industry regulation.
Other researchers have also used another database, the Federal Regulation and State Enterprise (FRASE) index, which combines RegData’s industry-level regulation data with U.S. Bureau of Economic Analysis data on the relative importance of each industry to individual state economies. This tool allows experts to weigh the relative importance of federal regulations at the state level. For example, a FRASE index value of “1” indicates that a state faces the same restrictions as the 1997 national average—the base year used for relative comparison. Values above “1” indicate an above-average regulatory burden, and values below “1” indicate the opposite.
Using FRASE in a 2019 study, Patrick McLaughlin, Laura Stanley, and I reported the impact of federal regulations on poverty rates across the states. We found that a 10 percent increase in a state’s federal regulatory burden as measured by the FRASE index is associated with a robust and statistically significant 2.5 percent increase in a state’s poverty rate—even when controlling for other explanatory factors common to the poverty research literature.
Given that higher federal regulations are associated with greater numbers of households living in the lowest income strata, it is no surprise that in a recent study, Colin O’Reilly and I found that higher levels of federal regulation are also associated with increased income inequality.
Using a dataset covering the period between 1997 and 2015, we found that a 10 percent increase in the FRASE index is associated with a 0.5 percent increase in state-level inequality as measured by the Gini coefficient—a common statistical measure of economic equality. While this may appear economically insignificant, if states are ranked in ascending order by income inequality—that is, from best to worst—such an increase in the Gini coefficient typically results in a two-position decline in a state’s inequality ranking.
With continued growth in the field of policy analytics and new quantitative measures, doors will open for economists seeking to study questions of economic inequality, and many more areas of economic regulatory inquiry that were previously siloed can be connected through machine learning metrics.