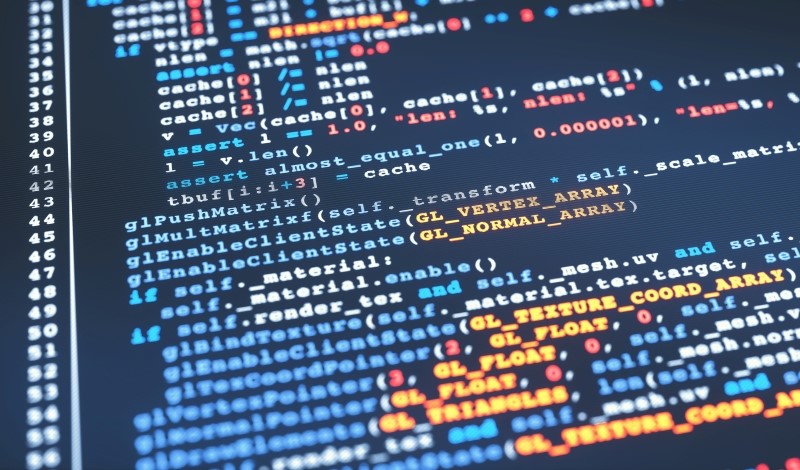
Scholar proposes mandatory self-audits and certification of employers’ hiring algorithms.
Several years ago, Amazon piloted an automated hiring system that, after some time, taught itself to discriminate against women. The company shut down the algorithm after learning that it had downgraded resumes that contained words such as “women’s” or indicated that the candidate had attended two specific all-women’s colleges.
Biased employment systems like the one that Amazon tried are on the rise in the wake of increased use of hiring algorithms, according to Ifeoma Ajunwa of the University of North Carolina School of Law. In a recent article, Ajunwa argues that hiring algorithms may violate antidiscrimination laws and run afoul of equal opportunity principles. Ajunwa further argues that regulators ought to require that employers perform routine audits of these tools.
Hundreds of the largest companies in the United States now use algorithmic hiring systems. These companies frequently defend their use of automated hiring systems by arguing that they are more objective than the human-driven alternatives, explains Ajunwa. But companies have other incentives for supplanting old, human-led decision-making tools: The new systems can cut the costs of recruitment and hiring, promote efficiency, and respond to a “talent scarcity” due to recent low unemployment.
But Ajunwa warns that the trend in using algorithmic hiring systems stems from “a misguided belief in the objectivity of automated decision-making.” The reality is that these algorithmic tools make decisions that resemble the biased inputs they receive from human-designed sources, argues Ajunwa. She states that they cannot be “fully disentangled from human decision-making.”
Ajunwa notes that employers tend to shield the workings and outputs of their automated hiring systems from the public eye. These systems often screen out applicants from backgrounds protected by antidiscrimination laws before even making a record of their application.
A job form, for example, might only allow for the entry of college graduation dates going back to the year 2000, which means that older applicants cannot fully complete online applications. These online forms mean that an employer can effectively reject a candidate on the basis of age—in potential violation of age discrimination laws—without preserving a record of its automated system’s unofficial “decision” on that applicant.
The implications of these discriminatory automated hiring processes for workers are significant, argues Ajunwa. Not only do workers lose out on some jobs due to biased automated decisions, but they also risk becoming “algorithmically blackballed.” That is, because employers retain increasingly large volumes of data from applicants, the same workers may experience repeated hiring discrimination, including by other employers, Ajunwa explains.
She argues that it is challenging for workers to ensure that employers’ hiring systems comply with equal opportunity principles. In particular, workers face obstacles in holding employers legally accountable for such biased decisions because hiring algorithms can often be proprietary and opaque.
As a result, in lawsuits under the U.S. civil rights statute known as Title VII, workers struggle to show their employers’ intent to discriminate, which they must prove to succeed on a disparate treatment argument. And if workers bring disparate impact claims under Title VII, they similarly face, in Ajunwa’s words, “an uphill climb.” Collecting the information from these hiring systems necessary to prove that their employers’ practices disproportionately affect protected groups, such as women and Black job applicants, is difficult.
Ajunwa argues that regulation requiring companies to audit their automated hiring systems to reduce bias and discrimination would be more effective at holding employers accountable than worker-led litigation. Specifically, Ajunwa recommends that legislatures mandate that companies conduct self-audits that confirm that their algorithmic hiring system is making accurate predictions.
Under Ajunwa’s mandatory self-audit proposal, employers would also have to separate demographic information on candidates’ race, gender, and age from the rest of a worker’s job application before passing the application on to the next decision-maker. Retaining this demographic information in a segregated database would allow regulators to measure disparate impact without letting those sensitive datapoints influence employers’ decisions, Ajunwa suggests.
Ajunwa envisions that self-audits would work best if they operated in conjunction with third-party auditors. For this reason, Ajunwa further proposes that a government agency serve as an external auditor of companies’ algorithmic decision-making tools. Ajunwa suggests that the U.S. Equal Employment Opportunity Commission could serve this role, providing its own auditing and certification of automated hiring systems before companies deploy them.
Alternatively, Ajunwa advises the U.S. Congress to relegate oversight of the auditing process to a non-governmental entity that would scrutinize and certify companies’ automated hiring practices. Such a third-party auditor could also help collect information from companies that might reduce some of the asymmetry between what workers and employers know about their automated hiring systems.
In Ajunwa’s view, mandatory auditing would work best with collective bargaining by workers to promote fairness in hiring and data protections that prevent algorithmic blackballing. Negotiations between labor unions and companies would be a valuable alternative to internal and external audits, Ajunwa argues.
Ajunwa concludes that automated hiring is a type of Trojan horse: although it saves employers time and money, “it may conceal amplified bias and replicate unlawful discrimination, all disguised as artificial intelligence.” She recommends self-auditing and third-party oversight as key measures to ensuring equal employment opportunities in an age of hiring by algorithm.