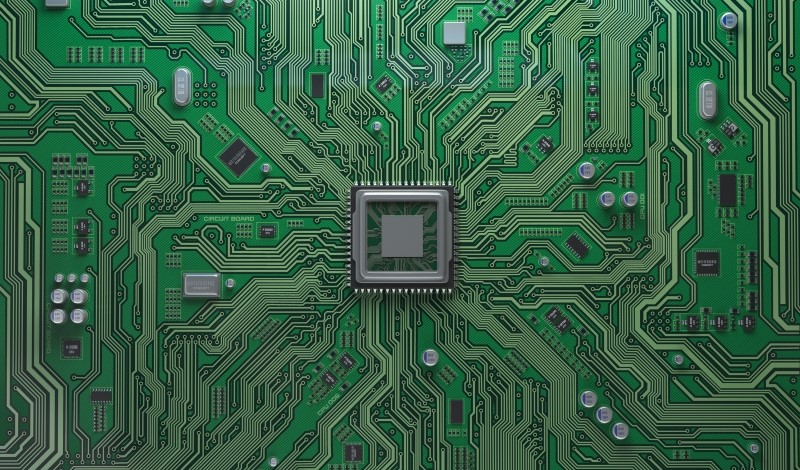
The rise of artificial intelligence has ushered in new regulatory opportunities and challenges.
The digital revolution and the innovations it has brought us in terms of speed, storage, and capacity—the very same technical innovations that put powerful computing in your pocket—have forever changed our economy. And it will require shifting regulation beyond a focus just on paper and people.
Most economic payments are now made computer to computer. Data are in. Paperwork is out. Paper has been disintermediated. Paper has disappeared. This is true with how people conduct almost all financial transactions. You use your phone to pay for groceries. You Venmo money to a friend. I recently traveled to Singapore to attend a meeting on global audit quality, and I never used cash or had to change money.
People also have been disintermediated. I can perform economic transactions without ever interacting with another human being. I can purchase stock on a variety of apps and never interact with a human being.
Algorithms push certain products towards me based on my past viewing or spending patterns. Although a human data scientist might have designed the original algorithm, no human is interacting with me in deciding what is pushed to my screen. In effect, interactions between humans are almost nonexistent.
All this disrupts a regulatory paradigm that concentrates on people and paper. It makes it quite challenging when thinking about regulatory incentives and disincentives or so-called carrots and sticks. How does a regulatory system provide incentives and disincentives? How does it gather facts without being able to examine human conduct and a paper trail? How does it pivot to a different way of operating?
And these questions are only more acute in today’s era of artificial intelligence (AI). Interest in AI exploded late in November 2022 with the release of OpenAI’s text-writing tool, ChatGPT. The technology underlying ChatGPT has also been incorporated into Microsoft’s Bing chatbot. And Alphabet Inc.’s Google has released its own AI contender, Bard.
Alan Turing, the British mathematician who devised the method for breaking the central “Enigma Code” used by Germany in WWII, is often thought to be the father of digital computers and of AI. Turing once said that “what we want is a machine that can learn from experience,” and he noted that the “possibility of letting the machine alter its own instructions provides the mechanism for this.”
In 1950, Turing devised a practical test for determining true AI. A human and a computer would respond to questions posed by a questioner. If the questioner could not distinguish the computer from the human, the computer would be considered an intelligent, thinking entity: artificially intelligent.
It may be that no machine or program has thus far fully passed the Turing Test. But it sure seems like we are close.
Machine-learning algorithms today have resulted in a variety of innovations: facial recognition and other visual authentication tools; Amazon’s Alexa and its tailoring of recommendations; widgets that provide the weather forecast on your mobile phone; robo-advisers; and even vaccine research. Through neural networks, self-improving algorithms, feedback-loops, and a few bells and whistles, the combination of complex algorithms and computer processing now appears intelligent in a human-like manner.
These AI technologies are and will have lasting impacts on both business and regulation. Could AI lead to untraceable market manipulation? To untraceable ways to launder money? To unheard of ways of accomplishing theft or fraud? No one yet knows. And we are just beginning to think of ways to create standards that can defend against such acts.
So, what could Regulation 2.0 look like?
Currently, most regulatory agencies have a narrow charge and a discrete number of tools to provide the public with benefits without creating any unintended effects. Agencies are also playing catch-up on the technological trends occurring in the private sector.
Innovations like ChatGPT, though, raise both opportunities and challenges.
For administrative agencies, the use of AI technology will likely bring new responsibilities. Thierry Breton, the European Union Commissioner for the Internal Market, has said that the upcoming EU Artificial Intelligence Act would include provisions targeted at generative AI systems, such as ChatGPT and Bard. Breton has explained that “AI solutions can offer great opportunities for businesses and citizens but can also pose risks. This is why we need a solid regulatory framework to ensure trustworthy AI based on high-quality data.” Agencies in Europe will be responsible for developing and carrying out that new regulatory framework.
Agencies will also confront the challenge of deepfakes. Algorithms may make it impossible to know what is real and what is not. As author Kirk Borne has observed, “AI has become so powerful, and so pervasive, that it’s increasingly difficult to tell what’s real or not, and what’s good or bad.” He has added that this technology is being adopted faster than it can be regulated.
Earlier this year, the nonprofit Future of Life Institute published a letter entitled “Pause Giant AI Experiments: An Open Letter.” It was signed by Elon Musk, Yoshua Bengio, Steve Wozniak, and other tech luminaries, and it argued for a six-month time-out so that basic safety rules can be created for the design of advanced AI. The authors of the letter stated that “these protocols should ensure that systems adhering to them are safe beyond a doubt.”
Whatever one makes of this open letter, or of what new protocols should say, a fundamental question must be confronted: How can regulatory agencies be more agile and nimble to deal with the opportunities and challenges created by digital innovations?
One way might be to make direct use of some of the same digital technologies that are advancing in the private sector.
In 1946, the Administrative Procedure Act (APA) demanded public participation in an administrative agency’s process of making rules. The APA requires public notice of a proposed rulemaking. Agencies must provide interested persons with a meaningful opportunity to comment on any proposed rule through the submission of written “data, views, or arguments.”
And yet, there is a widespread perception that in practice only sophisticated stakeholders, such as regulated entities, industry groups, law firms, and professional associations have the knowledge, time, and attention to contribute to the notice-and-comment process. The most important questions for agencies are: Who are we not hearing from? Who is not at the table?
In 2006, a feature article in Wired magazine described a trend in the way businesses were using the internet to collaborate on solving problems. Companies were posting problems on a website to elicit the help of “solvers”—that is, hobbyists and other members of the public. The article explained that “it’s not outsourcing; it’s crowdsourcing.”
Perhaps much the same ought to apply to administrative rulemaking. AI can radically improve the engagement process, in effect helping to crowdsource public involvement in the rulemaking process. For example, bots could target stakeholders and members of the public, explaining to them the problem underlying the proposed rule and what the potential regulatory solution would do. The same digital tools could provide easy ways for the public to submit comments too.
And as long as agencies have the requisite quality and quantity of data, they could use AI as a valuable tool in evaluating the efficacy of their existing rules—both qualitatively and quantitatively.
AI could help with enforcement too. Last December, the SEC announced fraud charges for a multi-year, multi-million-dollar front-running scheme. The SEC staff had analyzed trading using the consolidated audit trail database to uncover the allegedly fraudulent trading and to identify how an employee profited by repeatedly front-running large trades by his employer.
In a similar way, machine-learning algorithms could be used to analyze millions of records for patterns that may be indicative of misconduct. They could analyze trading patterns to assist with insider trading investigations. Machine-learning algorithms could also be used to triage complaints and tips.
The debate over the rules of the road will continue for years to come, as all of us become users of generative AI. Although regulatory agencies will not be able to afford or maintain the quality of technology of some of the most sophisticated market participants, algorithmic tools still have the potential to be great equalizers, even if government expertise and resources are constrained.
This essay is part of a three-part series based on remarks delivered at the Annual Distinguished Lecture on Regulation at the University of Pennsylvania Law School on March 29, 2023.
A fully formatted version of this entire three-part series is also available for download as a single, integrated PDF article.