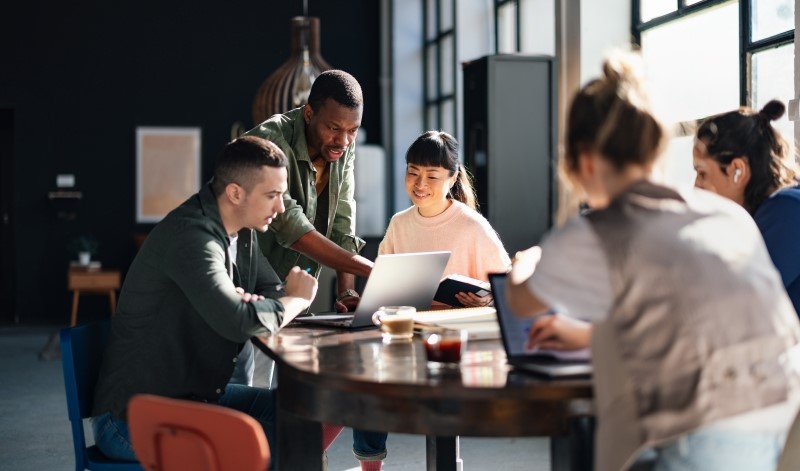
Scholars urge the European Union to take Artificial Intelligence Act enforcement seriously.
When you ask ChatGPT whether it would consider reactive or proactive governance to be more equitable, it responds: “it depends.” What ChatGPT does not emphasize is that it was one of the key technologies that influenced the European Union’s decision to shift from reactive to proactive AI governance, attempting to form a more comprehensive, principled vision for AI development.
In a recent article, Professors Oskar J. Gstrein, Noman Halleem, and Andrej Zwitter of the University of Groningen describe the recently passed European Union Artificial Intelligence Act (AIA), a hybrid regulation focused on safety and standardization of AI models while still granting some consideration to fundamental rights.
The authors present an initial analysis of the AIA, primarily emphasizing how critical effective enforcement will solidify it as the global benchmark for preemptive and proactive AI regulation.
The authors raise concerns about the logistics of enforcement that are currently being built up at both the national level of member states and at the EU level through a newly created AI Office. With AIA bans on “unacceptable risk” models and enforcement abilities against them becoming legally binding within a year of publication in the Official Journal, the official publication for EU legal acts, the authors argue that the AI Office might not be adequately staffed with trained experts and ready to operate by the time the regulations become enforceable.
The Act seeks to balance centralized and decentralized enforcement mechanisms. But the authors emphasize that critics fear that excessive enforcement power could end up being delegated to individual member states, either intentionally or because of limited resources at the international level. Given that EU member states vary in terms of priorities, AI literacy and skills, and access to resources, this could cause inconsistent enforcement from state to state. The authors caution against such a potentially uneven enforcement regime.
To overcome the decentralization problem and maintain equitable and even enforcement throughout the EU, the authors support the “development of sound administrative and market surveillance practices.” They emphasize the importance of the AI Office at the EU level being adequately staffed and integrated, “in quantity and quality of officials working there.” The authors also highlight the need to uphold democratic legitimacy in AI regulation. They warn that it could be undermined if unelected “technocrats” are responsible for interpreting the rules of the AIA across vast domains of AI usage, especially in various EU member states that lack the necessary expertise and resources to implement the regulations properly.
The rise of ChatGPT and similar AI chatbot systems in 2022 fueled debate among EU legislators drafting the AIA. While the AIA creates four risk categories for AI models, general-purpose artificial intelligence (GPAI) models are treated separately and are not assigned in any of these categories. The authors argue that AIA’s approach to regulating GPAIs makes GPAI regulation particularly dependent on careful enforcement. The authors note the regulations that govern GPAI models conflate the complexity of models and their function when categorizing them as GPAI and subsequently regulating them as such.
The AIA defines AI systems as those that operate autonomously, adapt after deployment, and generate outputs such as predictions, content, recommendations, or decisions. Foundation models (FMs), a type of GPAI, are trained on broad data and can be adapted to various tasks such as text and photo generation. The authors explain that FMs pose larger privacy concerns than other previous forms of AI models because they are more “data-centric,” a key reason for their separate treatment in the AIA.
While the AIA initially distinguished between GPAI and FMs, the final text does not. Article 53 of the AIA creates four obligations for GPAI system providers: publishing a training content summary, complying with copyright laws, sharing information with other providers, and providing documentation to oversight authorities. The authors note that the framework may be too broad for efficient and accurate investigations and enforcement, given the complexities of GPAIs covered.
GPAI providers face additional requirements if their models have been deemed to pose “systemic risk,” such as having a computational power greater than 10^25, an extremely advanced processing capability which allows models to deep learn from large data sets. Only the largest and most advanced FMs exceed that power. The authors suggest that this specific technical threshold is meant to allow for immediate investigation of the largest GPAI models, though the systemic risk classification remains “vague and complex.” The authors point out that the systemic risk classification will have to evolve based on the interpretation by regulators as enforcement begins.
The authors propose a three-tiered approach to categorizing the risks of general-purpose AI, rather than relying on a single systemic risk framework. Their approach seeks to remedy the shortcomings of the single systemic risk framework: unreliability and lack of transparency, dual-use issues related to the potential for AI to enhance cyber-attacks and exacerbate security risks, and systemic and discriminatory risks.