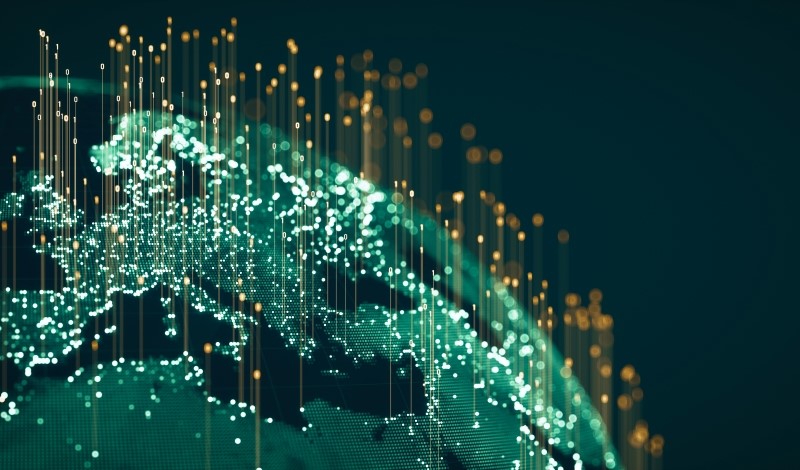
An overview of the Penn Program on Regulation’s year-long workshop series on “AI and Climate Change.”
In the race against climate change, artificial intelligence is the new kid on the block. But the early reactions are mixed. Some commentators see AI as the climate fight’s “secret weapon” while others are skeptical of its “energy guzzling” data centers.
To assess these varying viewpoints, the Penn Program on Regulation at the University of Pennsylvania organized a year-long workshop series centered on climate change and artificial intelligence. Built around presentations by six leading experts whose research cuts across a variety of disciplines, the workshop series illuminated both the risks and opportunities posed by this critical new juncture.
Climate scientist Tapio Schneider identified gaps in the “value chain” extending from climate data to information that is accessible and useful to end-users in the public and private sectors: one gap exists between the data and climate models, and another gap is between the models and end-users. Existing climate models are limited in their ability to capture fine-scaled, complex processes that are important for the earth system (such as clouds), leading to “divergence and inaccuracy in climate predictions.” By incorporating AI within climate models, scientists can combine the interpretability of existing physical models with deep learning’s capacity to represent complex processes, creating more accurate predictions without sacrificing interpretability.
Moreover, by leveraging AI-based emulators, climate scientists could establish an “ecosystem of apps” for end-users to access climate insights, generated on-the-go.
NASA statistician Amy Braverman addressed a similar opportunity for AI to improve the accuracy of climate forecasting through more complete use of the vast data collected by NASA. NASA’s recent advances in remote sensing technology are creating a vast repository of information that AI can harness for higher-resolution climate models. She advocated finding ways to keep these data sets from becoming relegated to what is “affectionately called the data morgue.”
Harvard law professor Cass Sunstein took a consumer-oriented focus on AI’s potential to mitigate the climate crisis. Central to his talk was the idea of AI-driven “choice engines,” which he described as algorithms that nudge consumers towards climate-friendly decision-making by addressing biases and information deficits. He argued that choice engines could reduce both the externalities of individual decisions—costs imposed on the climate—while also helping people better advance their own interests. He noted that such choice engines need necessarily be paternalistic—they could be simple and choice-preserving; they could take a “tell-me-everything” approach; or consumers could be asked which type of choice engine they want.
Of course, new innovations can come with risks. Professor Sunstein cautioned that if choice engines are not personalized enough, they may end up reproducing the problems of “mass interventions.” He also recognized that the risk could depend on the motives of the choice engine’s designer.
Media psychologist Asheley Landrum also examined another kind of AI risk related to climate change: its role in spreading climate misinformation. The rise of deepfakes and social bots suggests that AI poses an insidious threat to the media atmosphere that shapes public opinion. AI’s most immediate risk today seems to take the form of its impact on the credibility of information, creating anxiety that political actors are exploiting it to sow doubts and foster opposition to needed policy action.
Another key AI risk stems from its voracious appetite for energy. Penn Professor Deep Jariwala highlighted the rise in computing power required by AI and how this will place demands on memory-intensive hardware beyond production capacity. He cautioned that “the energy cost of AI will become unsustainable in about a decade or so unless energy policies and production of energy changes.” His co-presenter and fellow Penn Professor Benjamin Lee focused on how computing demand has driven a massive rollout of data centers, which are placing pressure on energy grids and leaving hefty carbon footprints.
To find a way toward carbon-free data centers, Professor Lee pointed to carbon-aware scheduling as a potential solution. Rather than simply “installing more, and more renewable, energy sources,” Lee argued for a demand-response approach. “You have to figure out how to schedule, how to modulate for computing, based on when renewable energy is more abundant,” he said. This flexibility could lower the operational carbon involved in computing, making the infrastructure that supports AI more climate-friendly.
McGill Professor David Rolnick urged taking a holistic approach to assess how existing social practices and paradigms that AI is shaped around—for instance, the use of AI to facilitate oil and gas exploration, or to drive digital advertising that increases consumption of resources—can also affect climate change.
“We need to think of AI for good,” Rolnick said. “It doesn’t mean just adding new good applications of AI on top of business as usual. It means shaping all applications of AI to be better for society.”